- Home
- Member Resources
- Articles
- Next Generation Anatomic Pathology: Innovative Imaging Technologies on the Horizon
Over the past 100 years, the diagnostic practice of anatomic pathology has been defined by information gleaned from manual evaluation of patient tissue sections through light microscopy. Recent advances and innovations in microscopic imaging technology are demonstrating the potential to significantly enhance the current clinical practice of diagnostic pathology. These techniques leverage unique data inherent to the tissue or tissue section, such as biochemical composition and autofluorescence properties. Novel methods have been developed to acquire microscopic images by utilizing fresh tissue and unstained tissue sections. Such diagnostic tools can generate microscopic images in real-time, provide a noninvasive alternative for obtaining definitive tissue-based diagnoses, and mitigate consumption of scant tissue samples. With techniques that do not require tissue sections, formalin fixation, or routine staining, a rapid preliminary diagnosis could potentially be rendered, and turnaround time could be significantly improved. This article is not meant to be a comprehensive review but rather aims to provide an overview of select emerging imaging tools that demonstrate how the future diagnostic practice of pathology could be enhanced.
Stimulated Raman Scattering Microscopy
Stimulated Raman scattering (SRS) microscopy is an emerging imaging technique that utilizes the vibrational properties of fresh tissue to generate microscopic images at a subcellular spatial resolution. Irradiating the unprocessed tissue sample with a light source generates a vibrational “fingerprint” that reflects its biological composition. Raman spectroscopic characteristics are altered by the presence of malignant cells, which can produce distinct spectral signatures due to differences in biochemical composition (e.g., fatty-acids, collagen, DNA and RNA). SRS microscopy can generate signals with high sensitivity to such differences, allowing for rapid microscopic imaging.1
An application of SRS microscopy using a portable fiber-laser-based microscope has been engineered for use in the operating room to provide intraoperative analysis of neurosurgical specimens.2 Using a supervised machine learning algorithm, the authors developed an image processing method--Stimulated Raman histology (SRH)--that rapidly converts images obtained by SRS microscopy of fresh brain tissue into hematoxylin and eosin (H&E)-like images in 2-3 minutes (FIGURE 1). These virtually stained H&E images demonstrated essential diagnostic histologic features that can differentiate different brain tumor types. Compared to smear preparation of brain tissue specimens, architectural information can be preserved given the minimal tissue alteration needed in SRS microscopy. SRH has been applied in the clinical setting to generate H&E-like images from fresh specimens for intraoperative diagnosis in over 1,000 patients.3 This technology has the potential to provide tremendous benefits with faster turnaround time, preservation of scant tissue specimen, and improved quality of digital microscopic images for remote evaluation by a pathologist.
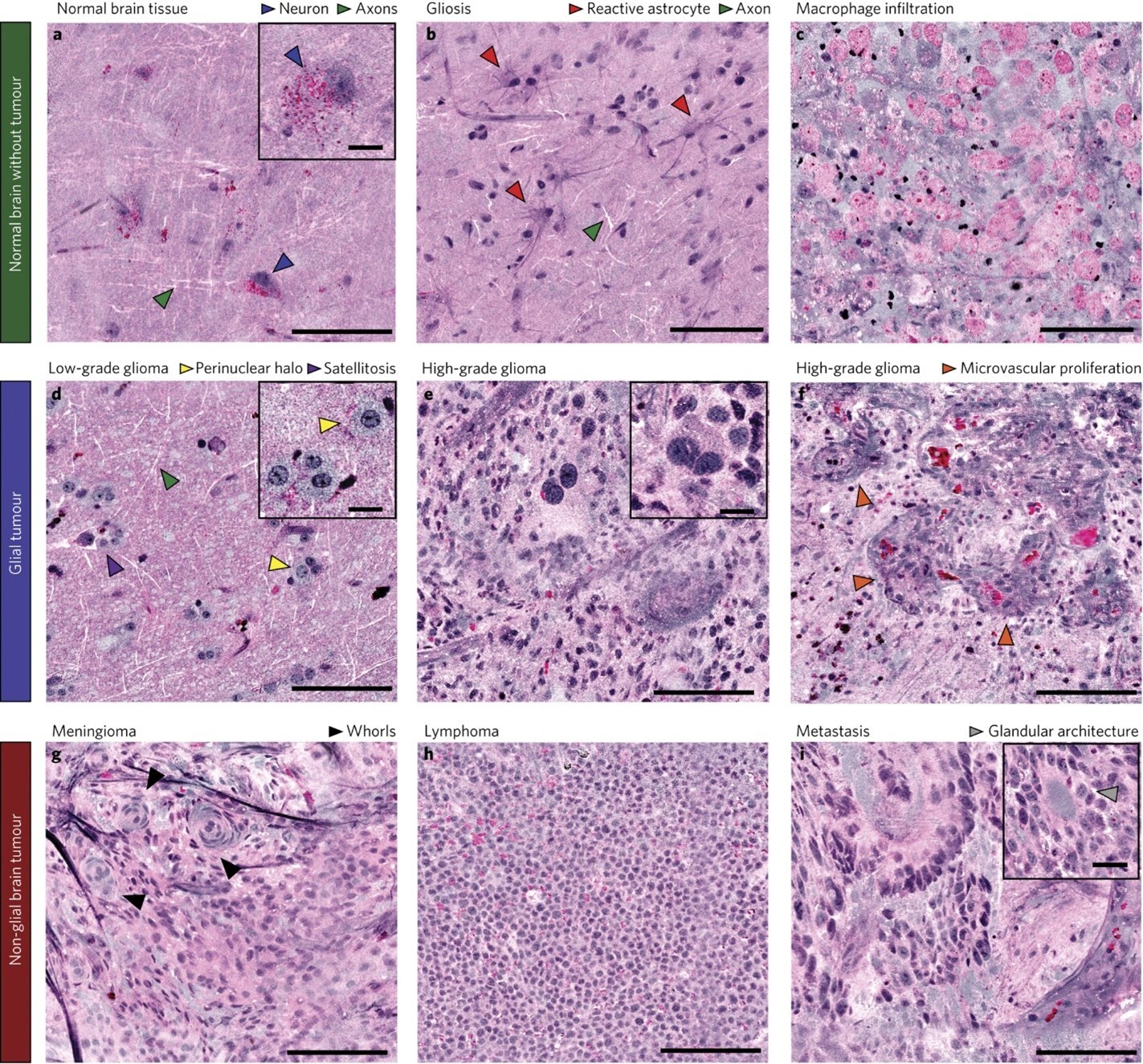
As a rapid, noninvasive imaging technique, SRS microscopy has the potential for in vivo clinical applications as an imaging modality that can generate microscopic images in situ without the need for tissue removal or processing. However, SRS microscopy is not yet widely used in the medical setting as SRS images can be heavily affected by background noise when imaging at a greater tissue depth or at a higher image acquisition rate. Efforts are underway to utilize deep learning methods to reduce background noise and improve quality of H&E-like images derived from SRS images.4
In Vivo Microscopy
Optical imaging technologies allow real-time visualization of microscopic images of tissue in patients (in vivo microscopy, IVM) or in freshly excised tissue (ex vivo microscopy, EVM). Optical imaging can generate high-resolution digital images of tissue without requiring tissue to be removed from the patient, formalin-fixed, processed, or stained. As optical images are similar to light microscopic images (FIGURE 2), pathologists are well positioned to interpret optical microscopy images.5 Examples of optical imaging instruments that are commercially available and US Food and Drug Administration-approved include optical coherence tomography and confocal microscopy.
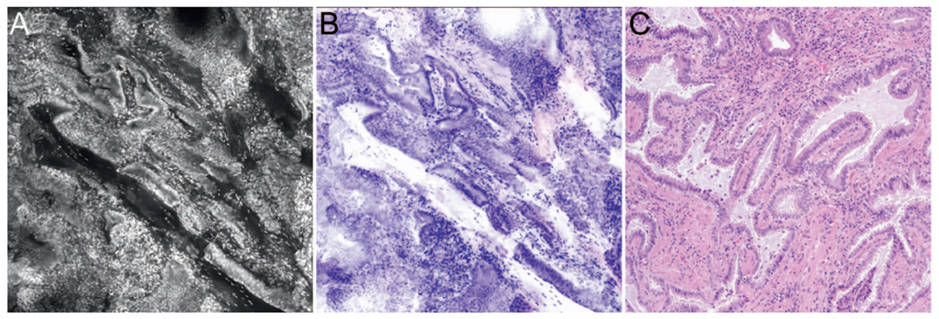
Optical coherence tomography can generate cross-sectional images with a near-cellular resolution (10 µm) in a matter of seconds up to a depth of 2-3 mm within tissue. This imaging modality measures the reflectance of near-infrared light and generates image contrast based on differences in endogenous tissue properties that lead to mismatches in the optical index of refraction. Superficial applications in ophthalmologic imaging allow detection of retinal disease and choroidal disease. Catheters have been designed for endoscopic applications in cardiovascular, upper/lower gastrointestinal, pulmonary, laryngeal, genitourinary imaging. Needle-based and laparoscopic catheter devices have been developed to image solid organs (e.g., breast, pancreas, lung parenchyma, kidney, ovary, and prostate). Swallowable capsule-based probes have also been developed for assessment of upper gastrointestinal tract lesions, such as Barrett’s esophagus.
Confocal microscopy can generate en face two-dimensional images at a higher, subcellular resolution (1-2 µm) up to a depth of penetration of 300 µm. This technology uses a pinpoint beam of light to generate image contrast from fluorescence emitted from the tissue, either from endogenous tissue autofluorescence or exogenous fluorescent dyes (injected intravenously or applied topically). A machine learning-based imaging processing algorithm can convert the grayscale images to pseudo-H&E images. Surface applications such as dermatologic imaging allow detection of pigmented skin lesions and margin assessment during Mohs surgery. Endoscope-based confocal imaging devices have been developed for luminal organ imaging (e.g., upper and lower gastrointestinal tract, bladder), and needle-based confocal imaging devices have been developed for solid organ applications.
Reflectance confocal microscopy (RCM) is currently used in clinical practice to diagnose pigmented skin lesions and CPT codes are available. RCM is considered the most clinically-validated optical imaging technology for identifying malignant dermatologic lesions with high sensitivity and specificity.6,7 As the images acquired through RCM are in grayscale, interpretation is challenging and requires specialized training. Recently, Li et al. (2021) demonstrated a deep learning-based framework to rapidly transform in vivo RCM images into virtually-stained H&E-like images at a microscopic resolution for normal skin, BCC, and melanocytic nevi.8 Such virtual histology staining methods will become increasingly valuable with the anticipated growth of clinical applications of in vivo microscopy in other organ systems.
As a noninvasive imaging modality that can rapidly generate microscopic images at the bedside, optical microscopy provides a significant added value for clinical diagnostics, particularly for close serial monitoring for dynamic pathologic processes, areas of the body prone to scarring, and patients with contraindications for biopsy. Artificial intelligence methods have been applied to optical microscopy images for real-time procedural guidance, screening, triaging, and preliminary diagnoses, and are increasingly being investigated for providing definitive diagnoses.
Numerous in vivo microscopy training and educational resources are available, including those provided by the College of American Pathologists (CAP).
Virtual Histology
As demonstrated above with confocal microscopy, endogenous tissue autofluorescence can be utilized to image tissue samples. Pseudo-H&E images can be created using endogenous fluorescence signatures, which harbor information related to functional and structural properties of the tissue. Deep learning approaches have been developed to generate virtually stained images from variety of image and tissue source types, including unstained formalin-fixed paraffin embedded (FFPE) tissue sections and grayscale in vivo RCM images.8,9 A recent study also demonstrated the use of deep learning methods to convert H&E images into special stain images (e.g., Jones, trichrome, periodic acid Schiff).10
Rivensen et al. 2019 developed a deep learning method to produce images of H&E and special stains from unstained FFPE tissue sections.9 In this study, unstained tissue sections were imaged with a fluorescence microscope using a near-ultraviolet light source. A deep convolutional neural network was trained to match autofluorescence images with their brightfield microscopic image counterparts (e.g., H&E, Jones, trichrome, periodic acid Schiff stain). This trained network was then used to generate a virtually stained image output corresponding to the input autofluorescence image of an unstained tissue section, bypassing the need for standard histochemical processing steps (FIGURE 3). These virtually stained images of H&E, trichrome, and Jones stains showed high degree of diagnostic agreement with conventional histology stains of the same tissue sections of salivary gland, thyroid, kidney, liver, and lung samples.
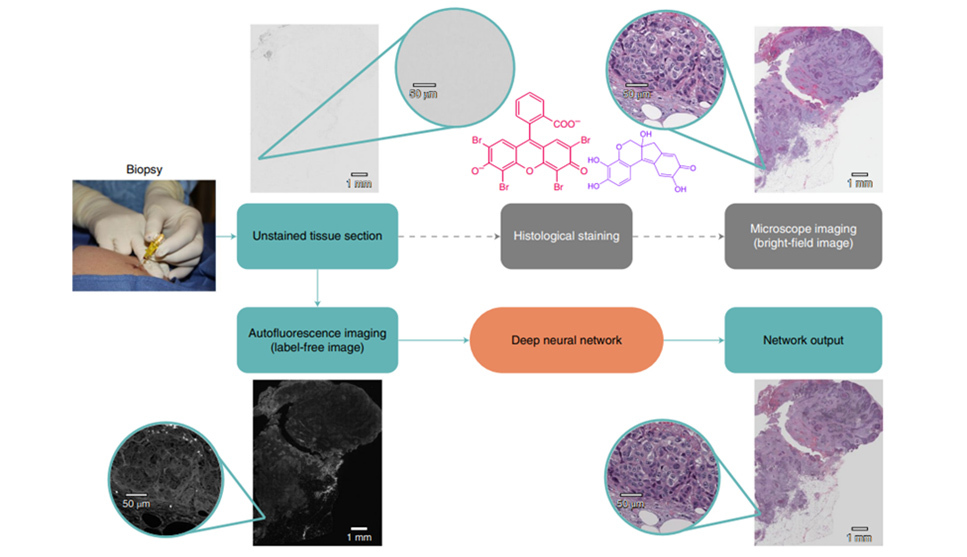
In Li et al. 2021, grayscale reflectance confocal microscopy (RCM) images of skin lesions were transformed into pseudo-H&E virtually stained images.8 In this study, a two-step approach was applied where the first trained deep neural network took a set of axially adjacent RCM images of a target lesion, converted them into a set of acetic acid virtually stained tissue images, which was subsequently transformed by a second trained deep neural network into H&E images representing serial sections of the lesional area. This method was used to transform in vivo RCM images into virtually stained, 3D pseudo-H&E images of normal skin, BCC, and pigmented melanocytic nevi that demonstrated diagnostic morphological features. To bring this technology to clinical adoption, efforts are currently under way to incorporate virtual histology with RCM images of a broader spectrum of skin disease, such as squamous cell carcinoma and actinic keratosis.10
As optical imaging technologies continue to advance, the demand for image processing methods that convert grayscale optical images into their counterpart conventional microscopic images can be expected to rise. Virtual staining in the ex vivo setting can offer numerous benefits as a method that does not consume tissue and is less susceptible to staining artifacts. Unlike conventional staining methods, the virtual staining approach can mitigate artifacts such as staining intensity variability and high non-specific background staining. In addition, scant tissue sections can be preserved for immunohistochemical stains or molecular analysis. Virtual staining eliminates time and resource intensive steps of histological staining as well as digitizing the glass slides, thereby improving turnaround time in the availability of digitized images.
Conclusion
Rapid advances are being made in emerging imaging technologies that have tremendous potential to drastically shift the paradigm of tissue-based diagnostics. The combination of powerful machine learning approaches and novel imaging techniques—like Raman spectroscopy and optical microscopy—allow biological data that are not currently visible through traditional pathology diagnostic methods to come to light. As the field of medicine trends towards noninvasive diagnostics, greater demand for interpretation of in vivo microscopic images can be expected in the future and pathologists are ideally positioned to interpret these images.
Some of the technologies discussed in this article have already been deployed in the clinical setting and demonstrate a number of areas in which the practice of pathology can be enhanced through novel imaging tools—through rapid assessment of fresh tissue without tissue consumption, real-time in vivo imaging of tissue, and improved turnaround time and quality of special stains. These tools shed light on rich information inherent to tissues that is not currently visible through conventional light microscopy. In combination with machine learning, advances in novel imaging modalities will continue to expand the armamentarium of diagnostic tools available to clinicians and improve the ability to provide personalized patient care.
References
- Saar BG, Freudiger CW, Reichman J, Stanley CM, Holtom GR, Xie XS. Video-rate molecular imaging in vivo with stimulated Raman scattering. Science. 2010 Dec 3;330(6009):1368-70.
- Orringer DA, Pandian B, Niknafs YS, et al. Rapid intraoperative histology of unprocessed surgical specimens via fibre-laser-based stimulated Raman scattering microscopy. Nat Biomed Eng. 2017;1:0027.
- Orillac C, Hollon T, Orringer DA. Clinical translation of stimulated raman histology. In: Ossandon MR, Baker H, Rasooly A, eds. Biomedical Engineering Technologies. Vol 2393. Springer US; 2022:225-236.
- Manifold B, Thomas E, Francis AT, Hill AH, Fu D. Denoising of stimulated Raman scattering microscopy images via deep learning. Biomed Opt Express. 2019 Jul 10;10(8):3860-3874.
- Wells WA, Thrall M, Sorokina A, et al. In vivo and ex vivo microscopy: moving toward the integration of optical imaging technologies into pathology practice. Arch Pathol Lab Med. 2019;143(3):288-298.
- Rajadhyaksha M, Marghoob A, Rossi A, Halpern AC, Nehal KS. Reflectance confocal microscopy of skin in vivo: From bench to bedside. Lasers Surg Med. 2017 Jan;49(1):7-19.
- Rao BK, John AM, Francisco G, Haroon A. Diagnostic Accuracy of Reflectance Confocal Microscopy for Diagnosis of Skin Lesions: An Update. Arch Pathol Lab Med. 2019 Mar;143(3):326-329.
- Li J, Garfinkel J, Zhang X, et al. Biopsy-free in vivo virtual histology of skin using deep learning. Light Sci Appl. 2021;10(1):233.
- Rivenson Y, Wang H, Wei Z, et al. Virtual histological staining of unlabelled tissue-autofluorescence images via deep learning. Nat Biomed Eng. 2019;3(6):466-477.
- de Haan K, Zhang Y, Zuckerman JE, et al. Deep learning-based transformation of H&E stained tissues into special stains. Nat Commun. 2021;12(1):4884.
- Borfitz D. " Virtual Histology Moving Closer to Clinical Adoption" Dec 14, 2021. Retrieved from: https://www.diagnosticsworldnews.com/news/2021/12/14/virtual-histology-moving-closer-to-clinical-adoption. Accessed 07/17/2022.
Iny Jhun, MD, ScD, is a Molecular Genetic Pathology Fellow at Stanford Health Care. Dr. Jhun has research experience in digital image analysis, molecular pathology, and public health.
Eric Walk, MD, FCAP, is chief medical officer at PathAI and a member of the CAP Personalized Healthcare Committee. He has more than 20 years of experience in pathology, oncology drug development, precision medicine, and medical device development.