- Home
- Member Resources
- Articles
- Revolutionizing Inflammatory Bowel Disease Histology Assessment Through Artificial Intelligence
Inflammatory bowel disease (IBD) is a cluster of primarily intestinal diseases, including Crohn's disease (CD) and ulcerative colitis (UC), which result from an uncontrolled immune-mediated inflammatory response in individuals with a genetic predisposition. Histological examination plays a crucial role in the diagnosis, classification, and management of IBD, providing valuable insights into disease severity, extent, and response to treatment.1-3 With the advent of artificial intelligence (AI) and machine learning (ML) techniques, there are many emerging applications in IBD with the potential to revolutionize patient care (Figure 1).
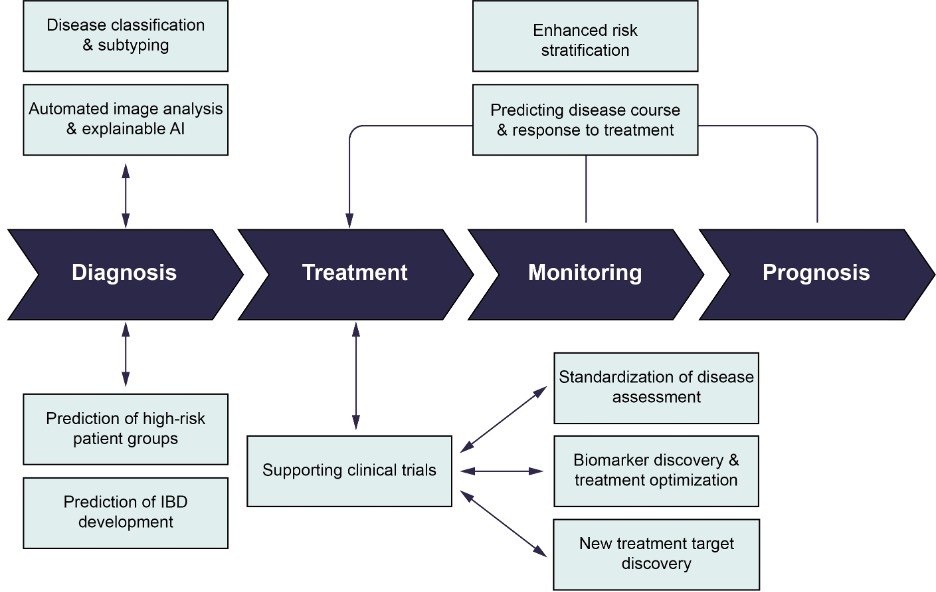
Automated image analysis and explainable AI
Traditional histological analysis in the context of IBD entails laborious visual examination of tissue slides by pathologists, rendering the process time-consuming and inherently subjective. To address these limitations, AI-based algorithms have emerged as a promising approach to automate histological analysis. By training ML models on extensive datasets comprising annotated IBD histological images, AI algorithms can acquire the capacity to discern and quantify specific histological features indicative of disease activity, such as inflammatory infiltrates and tissue damage.4 This automated analysis offers objective measurements, potentially enhancing the reproducibility of IBD histopathology assessment. Furthermore, AI-driven pathology effectively circumvents the challenges associated with manual quantification of cells in a biopsy. For example, cell classification models can proficiently label each cell in a whole slide image, enabling the differentiation of various cell types pivotal in the pathogenesis of UC, including neutrophils, plasma cells, and lymphocytes. The integration of cell classification and tissue segmentation models enables unparalleled spatial quantification of the immune microenvironment in colonic mucosal biopsies (Figure 2), thus serving as a potent research tool to unravel intricate tissue dynamics and gain deeper insights into disease pathogenesis.4
Subsequent investigations have employed comparable tissue segmentation and cell prediction techniques, utilizing interpretable features to construct a graph neural network capable of distinguishing normal colonic endoscopic biopsies.5 This approach not only optimizes the allocation of pathologist resources and reduces workload, but also contributes to early diagnosis facilitation.
Moreover, such methodologies hold potential as effective teaching tools for pathology trainees, functioning as personalized AI pathology tutors, facilitating a tailored learning experience for the next generation of pathologists. Additionally, these AI systems have the capacity to offer personalized feedback, further enhancing the educational value and proficiency development of trainees.
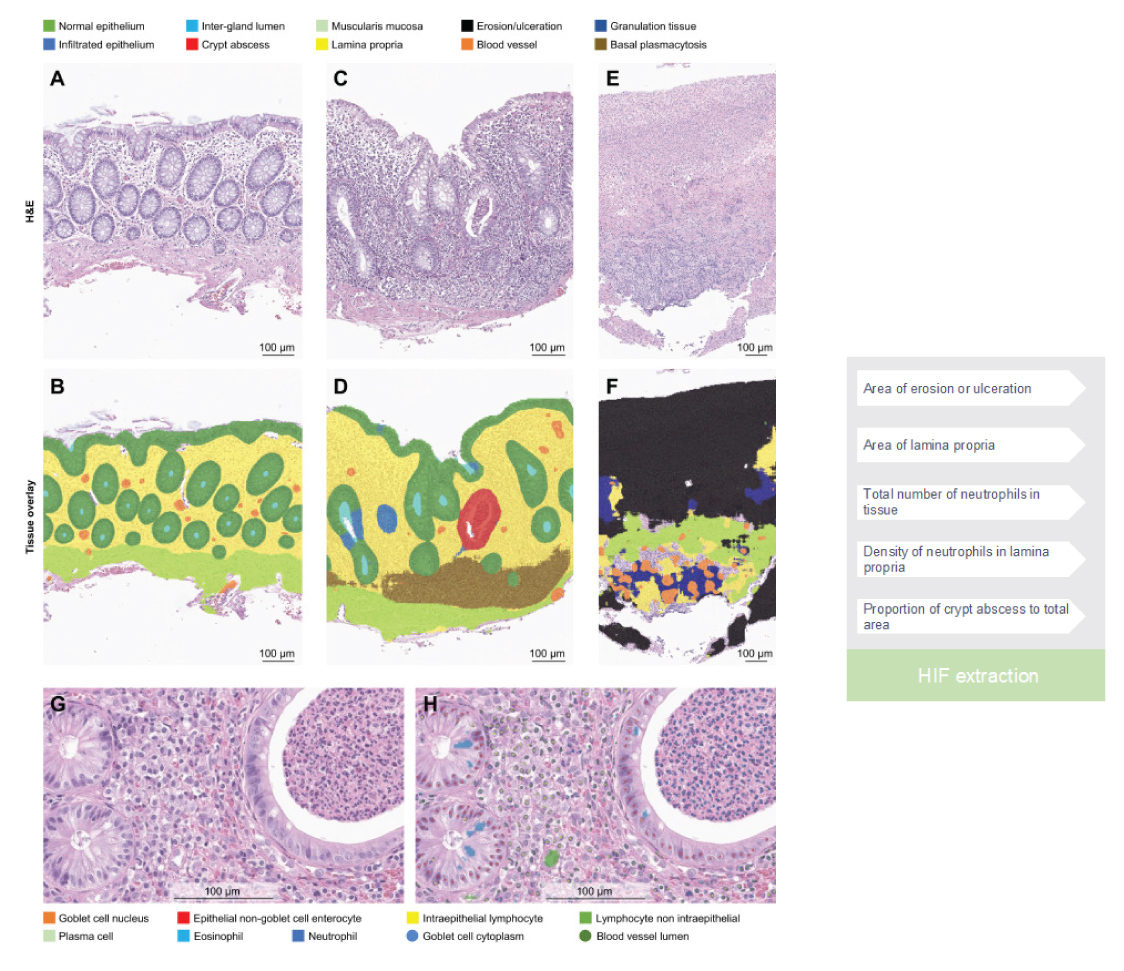
Disease classification
IBD encompasses a heterogeneous group of disorders, comprising distinct subtypes such as CD and UC. Accurate classification of these subtypes assumes paramount significance in guiding appropriate treatment strategies, particularly considering the challenges encountered in the pediatric population. In this context, AI algorithms have emerged as a valuable resource for automated disease classification, proficiently recognizing characteristic histological patterns associated with CD and UC while incorporating endoscopic findings in the training data.6
Predicting disease course and response to treatment
Forecasting the disease trajectory and treatment response in patients with IBD presents a complex yet imperative aspect of personalized healthcare. In this context, AI algorithms serve as a potent tool, capable of analyzing histological features to formulate predictive models. In a recent study, researchers demonstrated the capabilities of AI models in analyzing digitized biopsies from patients with UC to estimate the concurrent endoscopic activity and predict subsequent clinical outcomes.7 The potential implications of such predictive models are noteworthy, as they hold promise in empowering clinicians with a more comprehensive and data-driven framework to make informed decisions pertaining to tailored treatment strategies for individual patients.
Enhanced risk stratification
The identification of patients at heightened risk of disease complications, including strictures, perforations, or colorectal cancer, assumes critical importance in proactive disease management. In this context, AI algorithms emerge as a valuable tool, capable of analyzing histological features associated with disease severity and predicting the likelihood of disease progression and ensuing complications. For instance, AI models trained to predict p53 mutation from H&E-stained slides in cases of ulcerative colitis-associated dysplasia can offer an efficient and cost-effective digital workflow solution.8 Nevertheless, the utilization of such technology solutions mandates the prerequisite attainment of regulatory endorsement from the FDA.
By integrating diverse datasets encompassing clinical, genetic, and histological information, AI-based risk stratification models hold potential in identifying patients at high risk of adverse outcomes. This identification enables healthcare practitioners to tailor their approach, implementing more rigorous surveillance, early interventions, or escalated treatment strategies for individuals with increased vulnerability. By providing targeted and timely care to high-risk patients, AI-powered risk stratification fosters a proactive management approach, thereby enhancing patient outcomes and optimizing healthcare resource allocation.
Supporting clinical trials
In recent years, there has been an increasing recognition of the role of histology in clinical trials, with histology samples now routinely collected in clinical trials for UC. A growing body of literature is recognizing the value of histology, including the recent FDA guidance recommending the inclusion of histology as an exploratory endpoint. Three scoring indices were recommended by an international consensus to standardize the integration of histology in clinical trials.9-11 However, the absence of universally recognized endpoint definitions and a consensus pertaining to histologic response and remission remains a prevailing concern. This issue is further compounded by the reported interobserver variability among pathologists, which underscores the potential for AI-based solutions to introduce standardization in both the scoring system and the role of histology in disease evaluation, particularly in the context of clinical trials.12 Early endeavors in this domain have sought to recapitulate existing recommended scoring systems, such as the Geboes Score (GS) system, while others have focused on predicting remission versus disease activity.7,12-13 Moreover, some investigations have honed in on specific features, such as neutrophilic inflammation, to refine histological assessments.14 By harnessing the power of AI, these efforts aim to improve the consistency and reliability of histologic evaluations, fostering a more robust foundation for disease assessment in clinical trials and paving the way for enhanced precision medicine interventions for ulcerative colitis patients.
The development of new therapies with different mechanisms of action offers exciting prospects.15 However, it also highlights the need for precision medicine in the treatment of IBD, which demands a better understanding of biomarkers to identify subpopulations of patients who will best respond to specific medications. Histologic insights, aided by AI-powered analysis, have the potential to play an essential role in the research and clinical development space. Some early work in quantification of the immune microenvironment of IBD mucosal biopsies includes quantifying both cellular composition as well as tissue-level changes (Figure 2) that can characterize the inflammatory microenvironment with spatially-contextualized, single-cell resolution to unlock unprecedented insights from H&E and potentially identify novel histological biomarkers and drivers of therapeutic efficacy.4
Future vision
Foundation models, such as Generative Pre-trained Transformer 3.5 (GPT-3.5, OpenAI, San Francisco, CA), have demonstrated remarkable capabilities in natural language processing and understanding.16–20 These models can be harnessed as AI pathology general assistants to integrate multimodal data sources and up-to-date medical knowledge, providing valuable support in pathology decision-making. By analyzing diverse data inputs, including histopathological images, radiological data, and patient clinical information, foundation models can extract relevant insights and patterns, aiding in accurate disease diagnosis and severity assessment. Furthermore, these AI assistants can stay up-to-date with the latest medical literature, clinical guidelines, and research findings, ensuring that pathology decisions are informed by the most current knowledge. The ability of foundation models to synthesize information from multiple sources and their potential to assist pathologists in real-time decision-making makes them promising tools for enhancing pathology practice and improving patient care (Figure 3).
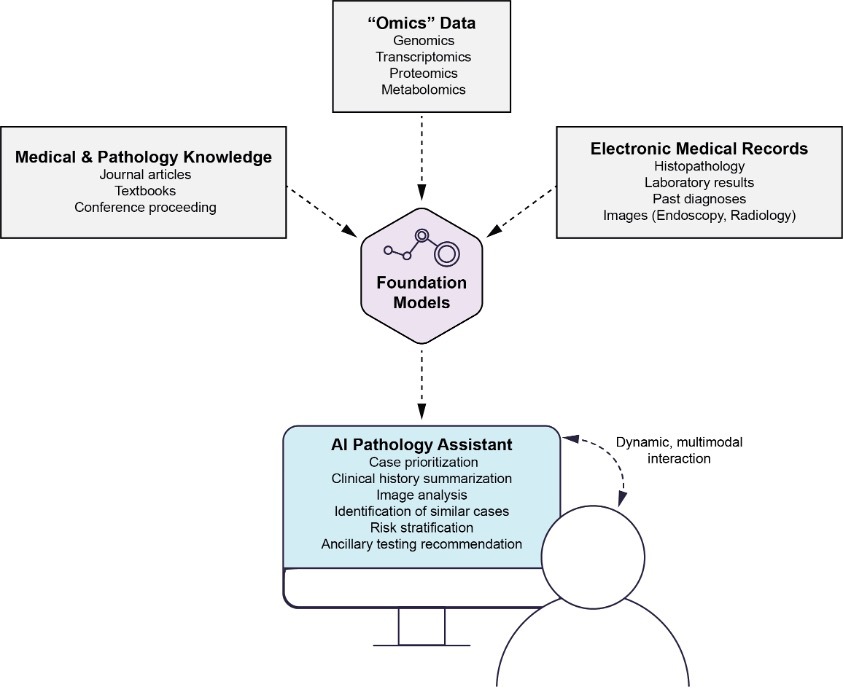
Conclusion
Artificial intelligence and machine learning techniques have the potential to transform the diagnostics of inflammatory bowel disease. Automated image analysis, disease classification, predictive modeling, and risk stratification are just a few of the emerging applications that can enhance diagnostic accuracy, improve patient management, and guide treatment decisions. However, it is important to acknowledge that AI algorithms should not replace the expertise of pathologists but rather complement their work, serving as decision support tools. As AI technology continues to advance, it holds great promise for optimizing patient care in IBD and improving outcomes for individuals affected by this complex disease.
While acknowledging the existing practical and ethical limitations and challenges in the integration of AI in pathology for IBD, coupled with the indeterminate reimbursement pathway for AI application in pathology by regulatory entities such as the Centers for Medicare & Medicaid Services (CMS) and insurance entities, this commentary aims to provide an enlightening overview of the immense potential that AI holds in this domain. The intention is to shed light on the transformative capabilities of AI, which, despite the challenges, offer promising prospects for improving IBD diagnosis, treatment, and patient outcomes.
References
- Khor B, Gardet A, Xavier RJ. Genetics and pathogenesis of inflammatory bowel disease. Nature. 2011;474(7351):307-317.
- Ungaro R, Mehandru S, Allen PB, Peyrin-Biroulet L, Colombel JF. Ulcerative colitis. Lancet. 2017;389(10080):1756-1770.
- Baumgart DC, Sandborn WJ. Crohn’s disease. Lancet. 2012;380(9853):1590-1605.
- Najdawi F, Sucipto K, Mistry P, et al. Artificial intelligence enables quantitative assessment of ulcerative colitis histology. Mod Pathol. 2023;36(6):100124. Reprinted under Creative Commons license BY-NC-ND 4.0
- Graham S, Minhas F, Bilal M, et al. Screening of normal endoscopic large bowel biopsies with interpretable graph learning: a retrospective study. Gut. Published May 12, 2023. doi:10.1136/gutjnl-2023-329512
- Mossotto E, Ashton JJ, Coelho T, Beattie RM, MacArthur BD, Ennis S. Classification of paediatric inflammatory bowel disease using machine learning. Sci Rep. 2017;7(1):2427.
- Iacucci M, Parigi TL, Del Amor R, et al. Artificial intelligence enabled histological prediction of remission or activity and clinical outcomes in ulcerative colitis. Gastroenterology. 2023;164(7):1180-1188.e2.
- Noguchi T, Ando T, Emoto S, et al. Artificial intelligence program to predict p53 mutations in ulcerative colitis-associated cancer or dysplasia. Inflamm Bowel Dis. 2022;28(7):1072-1080.
- Christensen B, Hanauer SB, Erlich J, et al. Histologic normalization occurs in ulcerative colitis and is associated with improved clinical outcomes. Clin Gastroenterol Hepatol. 2017;15(10):1557-1564.e1.
- Yoon H, Jangi S, Dulai PS, et al. Incremental benefit of achieving endoscopic and histologic remission in patients with ulcerative colitis: A systematic review and meta-analysis. Gastroenterology. 2020;159(4):1262-1275.e7.
- Ma C, Sedano R, Almradi A, et al. An international consensus to standardize integration of histopathology in ulcerative colitis clinical trials. Gastroenterology. 2021;160(7):2291-2302.
- Mosli MH, Feagan BG, Zou G, et al. Development and validation of a histological index for UC. Gut. 2017;66(1):50-58.
- Shanis Z, Padigela H, Sucipto K, et al. Machine learning\-based prediction of Geboes Score and histologic improvement and remission thresholds in ulcerative colitis. Gastroenterology. 2023;164(4):S25-S26.
- Gui X, Bazarova A, Del Amor R, et al. PICaSSO Histologic Remission Index (PHRI) in ulcerative colitis: development of a novel simplified histological score for monitoring mucosal healing and predicting clinical outcomes and its applicability in an artificial intelligence system. Gut. 2022;71(5):889-898.
- Cohen NA, Rubin DT. New targets in inflammatory bowel disease therapy: 2021. Curr Opin Gastroenterol. 2021;37(4):357-363.
- Brown T, Mann B, Ryder N, et al. Language models are few-shot learners. Adv Neural Inf Process Syst. 2020;33:1877-1901.
- LeCun Y, Bengio Y, Hinton G. Deep learning. Nature. 2015;521(7553):436-444.
- Liu Y, Ott M, Goyal N, et al. RoBERTa: A robustly optimized BERT pretraining approach. arXiv [csCL]. Published July 26, 2019. doi:10.48550/ARXIV.1907.11692
- Ronneberger O, Fischer P, Brox T. U-Net: Convolutional networks for biomedical image segmentation. In: Medical Image Computing and Computer-Assisted Intervention – MICCAI 2015. Springer International Publishing; 2015:234-241.
- Gulshan V, Peng L, Coram M, et al. Development and validation of a deep learning algorithm for detection of diabetic retinopathy in retinal fundus photographs. JAMA. 2016;316(22):2402-2410.
Eric Walk, MD, FCAP, is chief medical officer at PathAI and a member of the CAP Personalized Healthcare Committee. He has more than 20 years of experience in pathology, oncology drug development, precision medicine, and medical device development.
Fedaa Najdawi, MD, FCAP, is Director of Pathology at PathAI and a leader at the intersection of artificial intelligence (AI) and anatomical pathology. She guides a multidisciplinary team on a mission to improve patient outcomes with AI-powered pathology.
Dr. Najdawi's pathology journey commenced in Australia, where she embarked on her training in pathology. She honed her skills by completing two fellowships at Brigham and Women's Hospital, Harvard Medical School, specializing in gastrointestinal, liver, and endocrine pathology. With more than 13 years of experience in the medical field, she is certified to practice medicine in the United States, Australia, and Jordan.
Throughout her career, Dr. Najdawi has made significant contributions to the field, publishing and presenting at national and international meetings. Her current research is focused on leveraging AI and digital pathology tools for precision pathology. Some of her recent work includes AI applications in IBD, celiac disease, tumor microenvironment, and fibrosis analysis.